Integrating Revolutionary Scientific Advancements
The NeuroDetect Bioprofiler integrates three state-of-the-art approaches into one product:
▫ Human Induced Pluripotent Stem Cells (IPSCs) to obtain the patient blood sample.
▫ Microelectrode Array (MEA) to record cellular electrophysiology with laboratory precision.
▫ Genomics and other data to understand the patient’s systems biology.
Human Induced Pluripotent Stem Cells (IPSCs)
Neurons, astrocytes, and other cell types reengineered from IPSCs allow the complex modeling of the patient’s brain “on a chip.” Derived from a routine blood draw, IPSCs are a revolutionary and ethical approach to the development of patient-specific diagnostic biomarkers and precision-guided therapeutics. IPSCs are frequently integrated with other groundbreaking approaches, such as CRISPR, microelectrode arrays (MEAs), and the development of novel therapeutics.

Microelectrode Array (MEA)
An MEA is a laboratory device that records electrophysiology from neurons, astrocytes, and other cell types. The MEA chip is designed with a grid of hundreds to thousands of electrodes embedded at the bottom of the chip that measure cellular function, such as spike activity, network connectivity, and axonal conduction. MEAs are a high-throughput tool to develop diagnostic biomarkers and are emerging as a revolutionary method to develop precision-guided therapeutics.
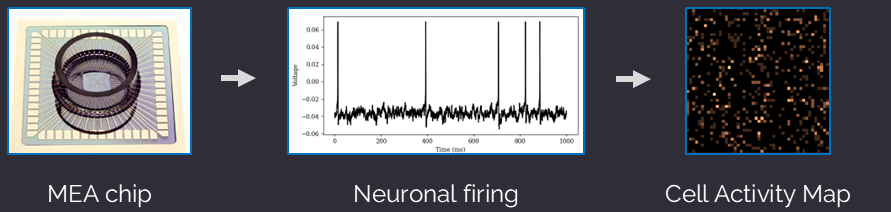
Genomics and More
Whole-genome sequencing and whole-exome sequencing have significantly advanced the diagnostic potential of laboratory approaches to genetic-based neurological disorders by establishing the cause in approximately 25% to 50% of cases. Other approaches, such as RNA sequencing, provide detailed functional characteristics of cell function.

Machine Learning
The NeuroDetect Bioprofiler utilizes a nonlinear statistical analysis module to create a patient-specific bioprofile. The validated biomarkers are passed to a neural network to provide a functional diagnosis, estimate of disease severity, and guide clinical decision-making for therapeutic recommendations.
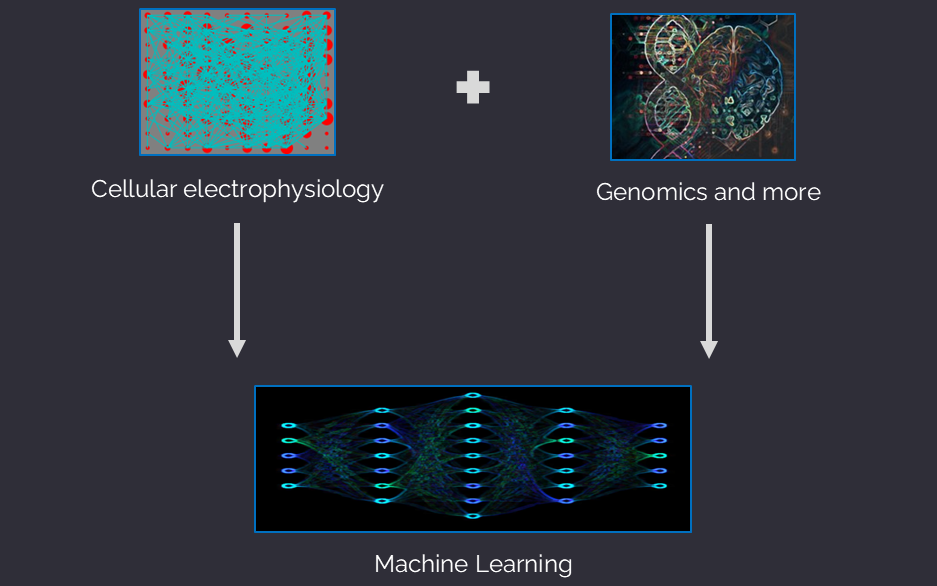
NeuroDetect Advancing Genomics, Neuroscience, and Beyond
Journal Article
Kress GT, Chan F, Garcia CA, Merrifield WS. Utilizing machine learning algorithms to predict subject genetic mutation class from in silico models of neuronal networks. BMC Med Inform Decis Mak. 2022 Nov 9;22(1):290.
Background
Genetic-based diagnoses have significantly advanced in the past decade due to access to next-generation sequencing and machine learning. Understanding how genetic function relates to cellular electrophysiology advances the state-of-the-art in the medical diagnosis of known and unknown genetic-based disorders. In silico modeling facilitates breakthrough diagnostics and development of therapeutics.
Methods
One second of data was simulated from neuronal networks cultured on a MEA. The predictive power of five machine learning approaches were tested to determine if the subjects were gain-of-function (GoF), loss-of-function (LoF), or healthy.
Results
By using only electrophysiological biomarkers from MEAs, machine learning algorithms were able to predict genetic mutation class in epilepsy with high accuracy.